How Has The Amazon Rainforest Changed Over Time?
Abstruse
The resilience of the Amazon rainforest to climate and state-use modify is crucial for biodiversity, regional climate and the global carbon bicycle. Deforestation and climate change, via increasing dry-season length and drought frequency, may already have pushed the Amazon shut to a critical threshold of rainforest dieback. Here, nosotros quantify changes of Amazon resilience by applying established indicators (for case, measuring lag-1 autocorrelation) to remotely sensed vegetation data with a focus on vegetation optical depth (1991–2016). Nosotros notice that more than 3-quarters of the Amazon rainforest has been losing resilience since the early on 2000s, consistent with the approach to a critical transition. Resilience is being lost faster in regions with less rainfall and in parts of the rainforest that are closer to human action. We provide directly empirical evidence that the Amazon rainforest is losing resilience, risking dieback with profound implications for biodiversity, carbon storage and climate change at a global scale.
Main
There is widespread business organization about the resilience of the Amazon rainforest to country-use change and climatic change. The Amazon is recognized as a potential tipping element in the World'south climate system1, is a crucible of biodiversitytwo and commonly acts every bit a large terrestrial carbon sink3,iv. The net ecosystem productivity (carbon uptake flux) of the Amazon has, even so, been declining over the terminal four decades and, during ii major droughts in 2005 and 2010, the Amazon temporarily turned into a carbon source, due to increased tree mortalityv,half dozen,7. Several studies have suggested that deforestationviii and anthropogenic global warmingix,10, peculiarly in combination, could push the Amazon rainforest past critical thresholdsxi,12 where positive feedbacks propel abrupt and substantial farther forest loss. Two types of positive feedback are peculiarly important. First, localized fire feedbacks amplify drought and associated forest loss past destroying trees13 and the fire regime itself may 'tip' from localized to 'mega-fires'xiv. Second, deforestation and woods degradation, whether due to direct man intervention or droughts, reduce evapotranspiration and hence the moisture transported further westward, reducing rainfall and woods viability in that locationxv and establishing a big-calibration moisture recycling feedback. Net rainfall reduction may in turn reduce latent heating over the Amazon to the extent that it weakens the depression-level circulation of the South American monsoon8. Model projections of futurity changes in the Amazon rainforest differ widely9,16,17,18. Early studies showed that the Amazon rainforest may exhibit strong dieback by the end of the 20-first century9,19. Both pronounced drying in tropical South America and a weak CO2 fertilization effect18 contributed to this result, with dieback also more common under stronger greenhouse gas emission scenarios17. Other studies based on varying general apportionment and vegetation model components show a wider range of resultstwenty,21. Nevertheless, the forest may be 'committed' to dieback despite appearing stable at the end of model runssixteen. This highlights the importance of measuring the irresolute dynamic stability of the forest aslope its mean land. Given the uncertainty in model projections, nosotros directly analyse observational data for signs of resilience loss in the Amazon.
The mean state of a system is not ordinarily informative of changes in resilience; either i can change whilst the other remains abidingsixteen,17. Thus, higher-order statistical characteristics that reply more than sensitively to destabilization than the mean need to be considered to quantify resilience. To measure the changing resilience of the Amazon rainforest, nosotros apply a stability indicator used to predict the approach of a dynamical system towards a bifurcation-induced disquisitional transition. The predictability arises from the phenomenon of critical slowing down22,23 (CSD): as the currently occupied equilibrium state of a system becomes less stable, it responds more sluggishly to curt-term perturbations (for example, weather variability for the Amazon). This loss of resilience, which is itself typically defined24 as the return charge per unit from perturbations, reflects a weakening of negative feedbacks that maintain stability. The behaviour can exist detected past an increment in lag-ane autocorrelation (AR(1)) in time series capturing the system dynamics25,26. It may also manifest as an increment in variance over time only variance can also be easily influenced by irresolute variability of the perturbations driving the system27. Increasing AR(ane) has been used to notice CSD before bifurcation-induced country transitions in a number of systems, including but not limited to climate25,28 and environmental29. In particular, CSD has recently been detected in reconstructions of western Greenland ice canvas height changes30 every bit well equally of the variability of the Atlantic Meridional Overturning Circulation31. A caveat, highlighted by analysis of model projections before Amazon dieback27, is that a system should be forced slower than its intrinsic response time calibration for CSD to occur (Methods). Hence, the absenteeism of CSD may not rule out the possibility of a forthcoming disquisitional transition. Conversely, increasing AR(1) tin sometimes occur for other concrete reasons. A space-for-time commutation has previously revealed that tropical woods resilience as measured by mean AR(1) (on a grid cell ground) is lower for less annual rainfall sums32 simply changes of Amazon resilience over time have not been investigated so far.
Nosotros investigate controls on the resilience of the Amazon vegetation system and how its resilience has inverse over the last iii decades, in terms of a changing AR(1) coefficient equally estimated from satellite-derived vegetation data. For comparison, we also investigate changes in variance over time, as a secondary indicator for CSD. The master dataset we employ is from the Vegetation Optical Depth Climate Archive (VODCA)33 but nosotros also analyse the NOAA Avant-garde Very-Loftier-Resolution Radiometer's (AVHRR) Normalized Departure Vegetation Index (NDVI)34 for comparison. Vegetation optical depth (VOD) has been previously used to estimate changes in vegetation biomass35, whereas NDVI is more commonly used to measure the greenness of vegetation (that is, photosynthetic action36), which can saturate at dumbo grass encompass. VOD, a microwave-derived production, does not saturate and remains sensitive to changes likewise at high biomass density35. Nosotros use the longer, Ku-band product from VODCA, which has a resolution of 0.25° × 0.25°, from which we create a monthly dataset past taking the hateful values and for direct comparing nosotros rescale the NDVI data to the same resolution. For a bank check of robustness, we also utilise the C-band production from VODCA, which spans a shorter time period. We focus on two stressors of the Amazon that may crusade resilience changes—atmospheric precipitation decline and human influence.
Results
Nosotros use the Amazon bowl as our report region and focus on those grid cells that have a ≥80% evergreen broadleaf (BL) fraction co-ordinate to the MODIS State Cover Type product in 200137 (Methods). Figure 1 shows that mean changes in BL fraction in this region correspond well to changes in VOD. Averaged across the Amazon study region we find overall decreasing VOD over 2001–2016, corresponding to the observed decrease in the number of filigree cells that have BL ≥ 80% each year (Fig. 1a). Betwixt 2001 and 2016, the BL fraction has changed near prominently in the s-eastern parts of the Amazon basin, forth parts of the Amazon River and in some northern parts of the bowl (Fig. 1b). Changes in VOD have a like spatial blueprint to changes in BL fraction, with decreases concentrated around the s-eastern edges of the forest (Fig. 1c). NDVI, in contrast, does not concur temporally or spatially with the changes in BL fraction (Supplementary Fig. ane), with NDVI increases observed in the south-eastern parts of the Amazon where deforestation rates are known to exist highest. On the individual grid cell level, changes in BL are strongly correlated with changes in VOD (Pearson'southward r = 0.556; Supplementary Fig. 2a) compared to changes in NDVI (r = −0.133; Supplementary Fig. 2b). This echoes previous in-situ comparisons betwixt VOD and NDVI38. Hence, we focus our analysis on VOD in the following, with results for NDVI in the Supplementary Figures.
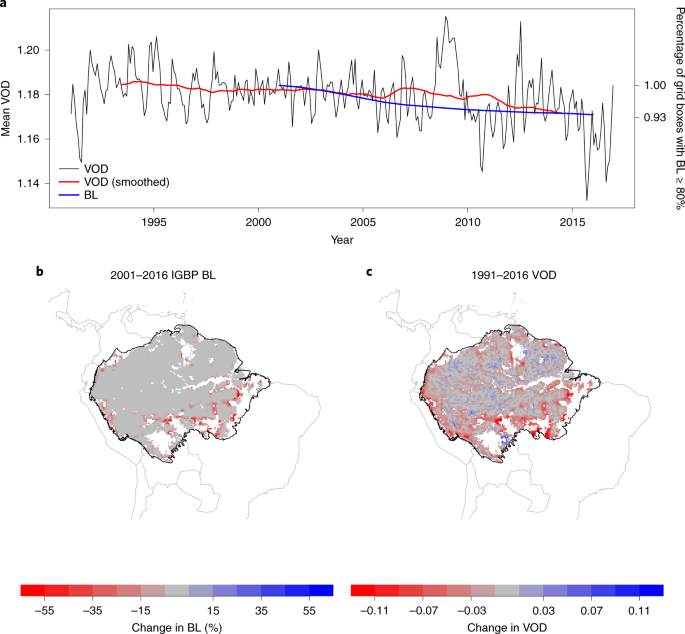
a, Fourth dimension serial of MODIS Country Cover evergreen BL fraction and VODCA Ku-ring product. Changes in BL fraction expressed equally the percentage of grid cells that have BL fraction ≥80% in each yr, compared to the number of grid cells that had BL fraction ≥lxxx% in 2001, and VOD is the monthly hateful. b, Changes in the BL fraction from 2001 to 2016 for filigree cells where the BL fraction is ≥80% in 2001. c, Changes in VOD from 1991 to 2016 (difference betwixt the 2012–2016 and 1991–1995 ways) for the grid cells where the BL fraction was ≥lxxx% in 2001. Country outlines were provided by the 'maps' package in R and Amazon basin outline was created from http://worldmap.harvard.edu/data/geonode:amapoly_ivb (Methods).
We brainstorm our resilience analysis by focusing on the temporal changes of AR(1), computed in sliding windows from the nonlinearly detrended and deseasonalized VOD fourth dimension serial (Fig. two and Methods). We remove forested (BL ≥ lxxx%) grid cells that have any human being state use in them (Methods), resulting in 6,369 filigree cells being analysed. The spatial distribution of the AR(1) trend, measured by the Kendall's rank correlation coefficient τ (Methods) at each grid prison cell, shows that the AR(1) increases in most of the grid cells comprising the Amazon rainforest (Fig. 2a,b). Likewise, the time serial calculated from the mean AR(ane) value across our report area each calendar month shows a substantial increase over fourth dimension, particularly from the early 2000s (Fig. 2c). Nosotros observe some stable or decreasing AR(one) values around the tributaries of the Amazon River, suggesting increasing resilience. VOD values can exist influenced past open water simply this should exist minimized by looking only at filigree cells where BL ≥ fourscore%. Previously, floodplain forests well-nigh the river, which cover fourteen% of the bowl, have been shown to exist much less resilient than the non-flooded forests throughout the Amazon39. All the same, when nosotros compare grid cells in the Amazon basin floodplains and those exterior (Methods), nosotros discover no significant differences between the resilience signals (Mann–Whitney U-test P = 0.579), except for a smaller Kendall τ value for floodplains from 2003 onwards (0.863 compared to 0.915; Supplementary Fig. three).
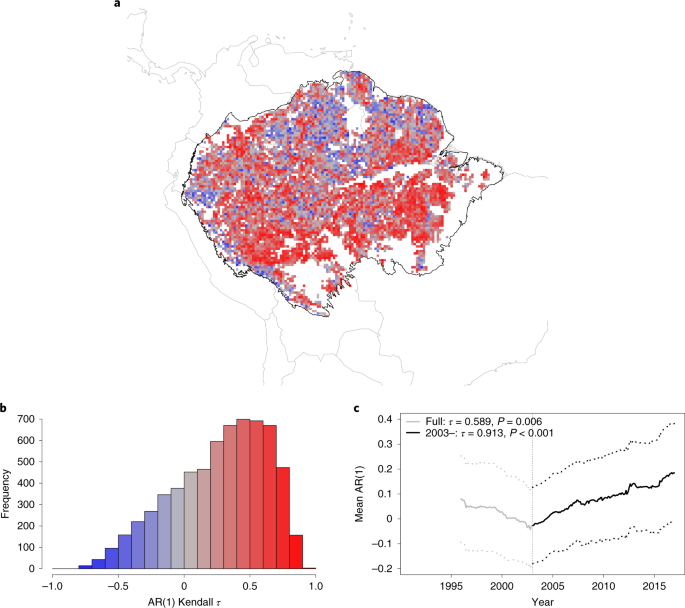
a, A map of the Kendall τ values of individual grid cells from 2003. b, Histogram of the Kendall τ values for the Amazon rainforest, considering data from 2003 onwards. Of the filigree cells, 76.2% have a positive Kendall τ value from 2003 onwards and 77.8% have this for the full time series. c, Mean VOD AR(i) fourth dimension series (solid line) along with ±1 due south.d. (dotted lines) created from grid cells that have BL fraction ≥80% in the Amazon bowl and likewise contain no human being land use (chief text and Methods). The full AR(ane) time series from 1991 (gray) has a Kendall τ value of 0.589 (P = 0.006) and from 2003 (black), a value of 0.913 (P < 0.001). Note that the AR(1) values are plotted at the stop of each 5-year sliding window. Land and Amazon basin outlines produced as described in Fig. 1.
Overall, most (76.2%) of the grid cells bear witness increasing AR(ane) values from the early 2000s onwards and hence, loss of resilience (Fig. 2b), as well equally 77.8% of grid cells over the full time menstruation. Using alternative methods of detrending the VOD time serial (Methods) yields like results (Supplementary Figs. 4 and 5), as does varying the window length used to guess AR(1) (Supplementary Fig. vi). The results are besides robust to the choice of the BL fraction threshold used to decide forest, finding increasing AR(one) too when either BL ≥ 90% or BL ≥ forty% is used (Supplementary Fig. 7). Furthermore, restricting the analysis to those filigree points that have BL ≥ 80% through the menses 2001–2016, rather than only checking filigree cells for BL ≥ 80% in 2001, shows similar signals of resilience loss (Supplementary Fig. eight). A predominance of increasing AR(one) trends is also establish for the NDVI fourth dimension series since 2003 (Supplementary Fig. ix).
To attempt and determine the causes for the detected resilience loss across the Amazon basin, we explore the relationships between the AR(1) trends and mean annual precipitation (MAP, estimated from the CHIRPS dataset40), as well as betwixt the AR(1) trends and the distance from human being country use (Methods). Information technology has previously been suggested that drier wood is less resilient32 equally well as wood nearer human land use41. We likewise include distance from roads alongside land use simply this restricts the domain of analysis to Brazil to avoid biases by heterogeneities in road data beyond dissimilar countries (Supplementary Fig. ten; Methods). Figure 3 compares the spatial patterns of the AR(i) trends, MAP and human land use. Relationships with both explanatory variables are discernible, with (less common) decreases in AR(1) (Fig. 3a) being establish in regions of high MAP in the northward of the region (Fig. 3b) and further away from human land utilise (Fig. 3c). We discover no misreckoning relationship between MAP and man land use; they are just very weakly correlated with each other (Spearman's ρ = 0.057, P < 0.001). Hence, we can consider them as separate relationships. The computed minimum distances to human country utilize and roads should exist interpreted every bit upper bounds because for the full region nosotros practice not include roads, and for the Brazil distances our dataset will not include non-federal or non-state roads, which also have human activity associated with them. Furthermore, the classification of filigree cells that contain human land utilize at the spatial resolution used in this analysis is likely to only observe large farms and settlements.
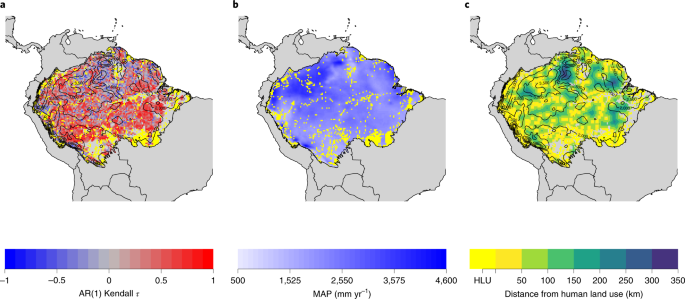
a, VOD AR(one) Kendall τ values (as in Fig. 2a). b, MAP from the CHIRPS dataset from 1991 to 2016. c, Distance from human being land apply (HLU) (Methods). In a–c, MAP contours are shown, along with HLU grid cells (yellow). Supplementary Fig. 10 shows the distance from HLU or Brazilian roads for the filigree cells in Brazil just. Country and Amazon basin outlines produced equally described in Fig. one.
To further explore the relationship between MAP and AR(i) trends, we create mean AR(1) time series on a moving MAP band of 500 mm (Methods). These bands evidence broadly the same behaviour as the region overall (Fig. 4a), with all bands showing a significant decrease in resilience post-2003 (P < 0.001 for all MAP bands). The increase in AR(ane) post-2003 appears least pronounced for the highest rainfall band (3,500–4,000 mm). The strength of resilience loss increases as the MAP band decreases beneath iii,500–4,000 mm (Fig. 4b). For NDVI, the same relationship is as well observed (Supplementary Fig. 11a,b). Withal, due to a large subtract in NDVI AR(1) pre-2003 beyond the region, analysing the full NDVI AR(1) time serial yield decreasing AR(one) Kendall τ coefficients for the college MAP bands.
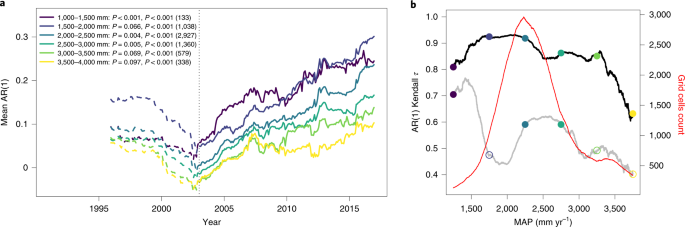
a, VOD AR(1) fourth dimension series for 500 mm MAP bands from 1996 (including data going dorsum to 1991; dashed lines) and from 2003 (including data going back to 1998; solid lines). The P values of the tendency significance test (Methods) are given in the legend; from 2003 onwards, they are all >0.001. b, VOD AR(1) Kendall τ series for a sliding MAP band with a width of 500 mm, from 1996 (grey) and from 2003 (blackness). Circles are coloured according to the corresponding time series shown in a and filled if the Kendall τ value is significantly positive (P < 0.05) and open up otherwise. The tendencies of the relationships in b are τ = −0.403 (grey) and τ = −0.463 (black), confirming at that place is a more astringent decrease in resilience with lower rainfall values. See Supplementary Fig. 15 for an uncertainty quantification of the results shown in b. The number of filigree cells used to summate the AR(1) time series and thus the Kendall τ values are shown in cherry in b. This never falls below 100 filigree cells and then we can exist confident in the mean estimation of the AR(one) time series. The number of filigree cells used in the adding of the time series in a is shown in brackets in the legend. Note that the AR(1) values are plotted at the end of each 5-yr sliding window.
To farther explore the human relationship betwixt resilience and the distance to man state apply, nosotros calculate mean AR(1) time series on fifty km altitude bands. Our results evidence that increases in AR(1) mail-2003 are stronger for grid cells closer to human state use (Fig. 5a). Above 200–250 km abroad from human state use the signal of loss of resilience becomes less pronounced (Fig. 5b). Using the subset of Brazilian filigree cells (n = 3,797) to include roads in our measurement of distance to human activity (Supplementary Fig. x; Methods) more often than not reduces distances merely we find a similar relationship, with decreases in Kendall τ coefficients observed up to 75 km (Fig. 5c,d). We notation in both cases that, at longer distances, the number of grid cells used to calculate the AR(one) time series is lower (ruddy lines and right-hand y centrality in Fig. 5b,d). The remaining areas also tend to become more than separated (the two vertical lines in Fig. 5b,d mark where <100 and <50 grid cells were left for the calculations, respectively). This helps to explain the more variable AR(ane) fourth dimension serial at greater distances (for example, the xanthous line in Fig. 5a) and the more fluctuating results for Kendall τ coefficients at greater distances (Fig. 5b,d). NDVI fourth dimension series also show a loss of resilience from the early on 2000s, in grid cells that are closer than 200 km from homo state use (Supplementary Fig. 11c,d). We reiterate that the stated minimum distances to human land use should be viewed as upper limits with, for instance, selective logging and other intrusions expected to be closer to the wood than the grid cells with major man land use and major roads. Comprehensive robustness tests, using alternative datasets and indicators, are presented in the Methods. In particular, nosotros notice overall consistent results when using the variance instead of the AR(1) as measure of resilience.
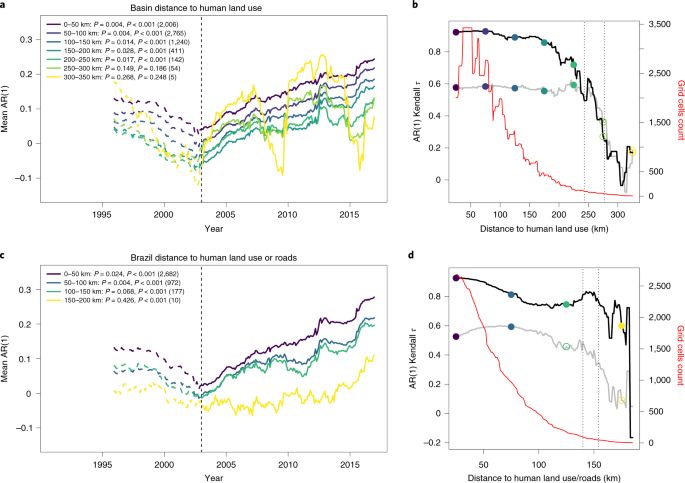
a, VOD AR(1) time series for l km bands measuring the minimum altitude a forested filigree prison cell is from a grid cell with human land use (divers in the Methods from the MODIS Country Cover product), from 1996 (dashed lines, these include data going back to 1991 due to the 5-yr sliding windows used to estimate the AR(ane)) and from 2003 (solid lines, including data going back to 1998), with the significance of these respective tendencies shown in the fable (Methods). b, VOD AR(1) Kendall τ series for the sliding 50 km bands, from 1996 (grey, again including data going back to 1991) and from 2003 (black, including data going dorsum to 1998). Circles are coloured according to the corresponding time series in a and are filled if the Kendall τ value is significantly positive (P < 0.05) and open otherwise. The tendencies of these relationships are τ = −0.574 (grey) and τ = −0.858 (black), showing that at that place is a more than astringent decrease in resilience with increasing proximity to human land use. The number of grid cells used to calculate the AR(ane) fourth dimension serial and thus the Kendall τ values are shown in red in b, with vertical dotted lines denoting where there are 100 and 50 filigree cells bachelor for the calculation. The number of grid cells used in the adding of the time serial in a is shown in brackets in the legend. c,d, The same as a and b, respectively, but for the subset of grid cells in Brazil, where reliable road information are available (as shown in Supplementary Fig. 10). For this case, where the distances from any given forested grid cell to human land use or roads are computed, the trends in the Kendall τ serial are τ = −0.688 and τ = −0.679, respectively.
Give-and-take
We reiterate that changes in the mean state of a organization practise not direct chronicle to changes in resilience. Model studies prove that large parts of the Amazon rainforest can be committed to dieback16 before showing a strong change in mean state. Indeed, from our CSD indicators nosotros infer a marked loss of Amazon rainforest resilience since the early 2000s, in vast areas where the BL fraction has non strongly decreased (compare Figs. 1b and 2a or Supplementary Fig. 8b).
Given that lower baseline MAP (Fig. four) and greater proximity to human interference (Fig. 5) are both statistically associated with greater loss of resilience, we hypothesize that depression MAP and increasing human interference could both be contributing to the large-scale loss of resilience (Fig. 2). What remains to be explained is why these two factors might play such an important function and why the large-scale resilience loss started in the early 2000s.
Previous work32 has shown that regions with lower MAP have lower absolute forest resilience, conceivably because vegetation is more water stressed and struggles to regulate its internal water content. VOD, being dependent on this h2o content, would consequently suit more than slowly to perturbations. Our finding that resilience has been lost faster in lower MAP regions, additionally suggests that vegetation in regions with more pronounced aridity stress is at greater risk of losing resilience. Large parts of the report region show decreasing MAP. Nonetheless, the spatial pattern of MAP change (as measured by the difference between the means for January 1998 to December 2002 and January 2012 to December 2016; Supplementary Fig. 12) is dissimilar to that of AR(1) increases (Fig. 2) and nosotros find no spatial correlation between these MAP changes and VOD AR(1) Kendall τ (Spearman's ρ between the spatial field of MAP change and the spatial field of VOD AR(1) Kendall τ equals 0.04). Increases in dry out-season length (DSL; Supplementary Fig. 12) reported in several contempo studies42,43,44,45, might feasibly exist a better explanatory variable but once again nosotros find no spatial correlation between DSL alter and VOD AR(1) Kendall τ (ρ = −0.08). The lack of spatial correlations for both MAP and DSL could exist due to the relatively brusk period to measure rainfall trends and for DSL due to the discrete nature of DSL values, which are given in units of full months, compared to the continuous τ values.
Despite a lack of spatial correlations, existing understanding and larger-scale aggregate measures suggest that climate variability may be among multiple factors contributing to the observed Amazon resilience loss since the early on 2000s (Fig. six). Notably, the Amazon shows signs of resilience loss during a period with three 'ane-in-a-century' droughtsten,46,47,48. Bounding main surface temperature (SST) anomalies in the northern tropical Atlantic Bounding main (from the HadISST49 datatset; Methods) from around 2000 onwards accept been mostly positive compared to climatology, consistent with a shift of the Atlantic Multidecadal Oscillation (AMO) to its positive phase (Supplementary Fig. thirteen), although reductions in anthropogenic northern-hemisphere aerosol cooling may also play a role10. These positive northern tropical Atlantic SST anomalies led—via the associated northward shift of the Intertropical Convergence Zone—to drier conditions in the Amazon and, in particular, to two severe droughts in 2005 and 201046,48 (Fig. 6a). These two drought events are associated with respective peaks in the spatial-mean AR(1) time serial, superimposed on the overall positive trend (see arrows in Fig. 6b). These peaks are as well found in the separate AR(1) time series in Fig. 4a, actualization well-nigh 2.5 yr ahead due to the time serial being plotted at the terminate of the 5-year sliding windows used to summate the AR(1) in that location. Moreover, a 3rd, El Niño-driven drought in 2015/16 is accompanied by an increased overall charge per unit of resilience loss at the very finish of the time range for which the VOD data are available. The decrease in AR(1) earlier the early on 2000s may also be linked to internal climate variability; the AMO was in its negative phase (Supplementary Fig. 13), consistent with negative SST anomalies in the northern tropical Atlantic (Fig. 6) and wetter atmospheric condition in the Amazon. The fact that the AR(1) increment since the early 2000s is statistically strongly significant suggests that information technology is non just due to natural climate variability.
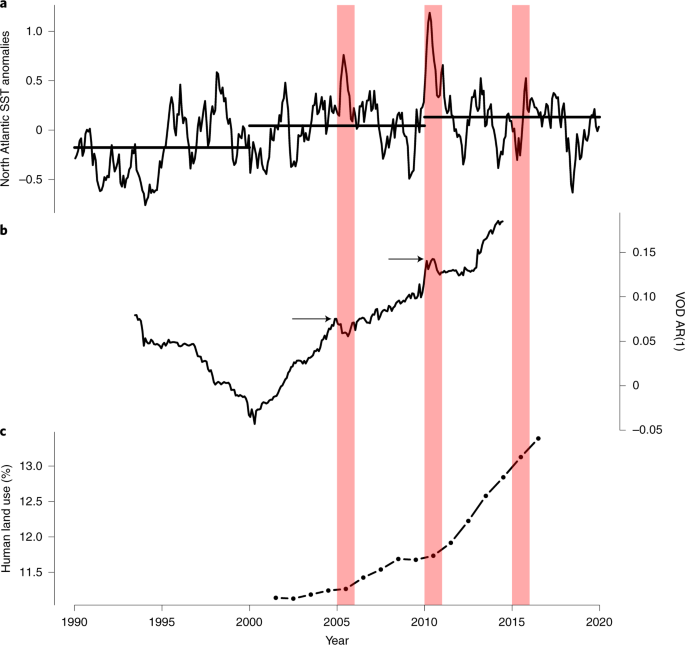
a, Northern tropical Atlantic SST anomalies averaged over 15–seventy° West, 5–25° N, once a mean monthly bike has been removed. Horizontal black lines denote the decade-mean anomalies. b, Spatially averaged Amazon bowl VOD AR(1) fourth dimension series as in Fig. 2, plotted at the midpoint of the window used to calculate AR(1) rather than at the terminate of the window. c, Almanac time series of percentage of filigree cells in the Amazon basin that have human being state use (as described in Methods). Cerise bands refer to 2005, 2010 and 2015, which were severe drought years in the Amazon basin. Arrows in b show the top value in AR(ane) in or near the drought years. These acme values may appear before due to the AR(1) time serial existence calculated on a moving window, compared to the SST anomalies beingness a monthly hateful.
Increasing human land utilize also appears to be contributing to the observed Amazon resilience loss, with human land-use areas increasing in both attain and intensity (Fig. 6c and Supplementary Fig. 14). Notably, the expansion of human land use accelerates after 2010, in an interval that also shows accelerated resilience loss (Fig. 6b) but less striking northern tropical Atlantic SST anomalies (Fig. 6a). Greater proximity to human state use tin increment disturbance factors such every bit directly removal of copse, construction of roads and fires, feasibly reducing absolute resilience (Fig. 5) and making the forest more prone to resilience loss.
Other factors, including rise atmospheric temperatures in response to anthropogenic greenhouse gas emissions, may additionally have negative effects on Amazon resilience (and are contributing to the warming of northern tropical Atlantic SSTs; Fig. 6a). Furthermore, the rapid change in climate is triggering ecological changes but ecosystems are having difficulties in keeping stride. In particular, the replacement of drought-sensitive tree species by drought-resistant ones is happening slower than changes in (hydro)meteorological conditions50, potentially reducing wood resilience further.
In summary, nosotros have revealed empirical testify that the Amazon rainforest has been losing resilience since the early 2000s, risking dieback with profound implications for biodiversity, carbon storage and climate change at a global scale. We farther provided empirical bear witness suggesting that overall drier conditions, culminating in three severe drought events, combined with pronounced increases in human land-use activity in the Amazon, probably played a crucial part in the observed resilience loss. The amplified loss of Amazon resilience in areas closer to human country utilise suggests that reducing deforestation will non just protect the parts of the wood that are directly threatened merely also do good Amazon rainforest resilience over much larger spatial scales.
Methods
Datasets
We use the Amazon basin (http://worldmap.harvard.edu/data/geonode:amapoly_ivb, accessed 28 January 2021) as our region of study. To decide the grid cells that are independent within Brazil for a subset of analysis, we use the 'maps' package in R (v.3.3.0; https://CRAN.R-project.org/package=maps). This is also used in the plotting of country outlines. The chief dataset used to determine forest wellness is from VODCA33, of which we use the Ku-band product. These information are available at 0.25° × 0.25° at a monthly resolution from January 1988 to December 2016. Nosotros too utilize NOAA AVHRR NDVI34. For precipitation data, we use the CHIRPS dataset40 downloaded from Google Earth Engine at a monthly resolution. Finally, to decide land cover types, we used the IGBP MODIS country cover dataset MCD12C1 (ref. 37). All these datasets are at a higher spatial resolution than the VODCA dataset and thus we downscale them to match the lower resolution. Our SST data comes from HadISST49, where we ascertain a North Atlantic region (15–70° W, 5–25° N), for which we take the spatial hateful. The mean monthly cycle is then removed to produce anomalies.
For the vegetation datasets that nosotros measure the resilience indicators on (below), we utilise STL decomposition (seasonal and trend decomposition using Loess)51 using the stl() function in R. This splits fourth dimension series in each grid cell into an overall trend, a repeating annual bicycle (by using the 'periodic' option for the seasonal window) and a residual component. We use the residual component in our resilience analysis. The beginning 3 yr of information had large jumps in VOD which were seen when testing other regions of the world every bit well as in the Amazon region. Hence, we restrict our analysis to the period Jan 1991 to Dec 2016.
To examination the robustness of the detrending, we as well vary the size of the trend window in the stl() function. The results from these alternatively detrended time series are shown in Supplementary Fig. four. The results are also robust to varying the window used to summate the seasonal component rather than using 'periodic'; at the strictest plausible value of 13, we nevertheless see the same increases in AR(1) (Supplementary Fig. 5).
For the AMO index shown in Supplementary Fig. thirteen, data come from the Kaplan SST dataset and can be downloaded from https://psl.noaa.gov/information/timeseries/AMO/.
Grid cell choice
Nosotros use the IGBP MODIS land cover dataset at the resolution described higher up to make up one's mind which grid cells to use in our analysis. The dataset is available at an almanac resolution from 2001 to 2018 (just nosotros just use the time series upward to 2016 to lucifer the fourth dimension bridge of our VOD and NDVI datasets). To focus on changes in forest resilience, we use grid cells where the evergreen BL fraction is ≥80% in 2001. Grid cells are treated equally human land-use area if the built-upwards, croplands or vegetation mosaics fraction is >0%. We remove grid cells that have human country apply in them from our forest analysis, regardless of if there is ≥80% BL fraction in the grid cell.
We measure out the minimum distance between forested Amazon basin grid cells and human land-use grid cells in 2016 (assertive this to be the almost cautious and least biased way to measure distance) using the breadth and longitude of each grid indicate and calculating the cracking-circle distance. We apply man land-use grid cells over a larger area than the basin, then that nosotros can make up one's mind the closest altitude to homo land utilise, regardless of whether this human country use lies within the basin. We as well measure the minimum altitude from homo land employ or roads in Brazil, where we have reliable data on state and federal roads (https://datacatalog.worldbank.org/dataset/brazil-route-network-federal-and-state-highways). As in the chief text, we reiterate that these minimum distances can be viewed as the maximum altitude from human being land use as our information volition not include roads for the full Amazon basin, or non-federal or non-state roads in Brazil that will take homo activity associated with them.
To ensure that the pattern of changes in resilience is not a consequence of more settlements being in the southeast of the region, combined with the gradient of rainfall from northwest to southeast typical of the rainforest, we measure the correlation between MAP and the distances from the urban grid cells, which is very weak (Spearman's ρ = 0.109, P < 0.001) and as such we are confident that there are carve up processes that causes these relationships.
Resilience indicator AR(1)
Nosotros measure our resilience indicator on the residual component of the decomposed vegetation time serial. We focus on AR(1), which provides a robust indicator for CSD before bifurcation-induced transitions and has been widely used for this purpose23,25,32. We measure it on a sliding window length equal to v yr (threescore months). The sliding window creates a fourth dimension serial of the AR(1) coefficient in each location. Our results are robust to the sliding window length used, every bit shown in Supplementary Fig. half dozen.
From linearization and the analogy to the Ornstein–Uhlenbeck process, it holds approximately that for detached time steps of width Δt (1 calendar month in the case at manus):
$$\mathrm{AR}\left( 1 \right) = \mathrm{east}^{\left( { - \kappa {\Delta}t} \correct)},$$
where κ is the linear recovery rate. A decreasing recovery charge per unit κ implies that the system's capability to recover from perturbations is progressively lost, respective to diminishing stability or resilience of the attained equilibrium state. From the to a higher place equation it is clear that the AR(1) increases with decreasing κ. The point at which stability is lost and the system will undergo a critical transition to shift to a new equilibrium state, corresponds to κ = 0 and AR(1) = 1, respectively.
Measuring AR(ane) across the whole time series provides information about the feature time scales of the two vegetation datasets we use26. Inverting κ gives the characteristic time scale of the system; for the VOD, we detect i/κ = one.240 months, whereas for the NDVI, we observe 1/κ = 0.838 months when using the hateful AR(ane) value beyond the region. This suggests that, in accord with our interpretation of the 2 satellite-derived variables, the NDVI is more than sensitive to shorter-term vegetation changes such as leafage greenness, while the VOD'due south Ku-band is sensitive to longer-term changes such as variability in the thickness of woods stems.
Creation and tendency of AR(1) and variance time series
For analyses where either MAP bands or distance bands are used to create an AR(1) or variance series, we calculate the mean AR(one) or variance value in each calendar month for forested (BL ≥ lxxx%), not-human country-employ Amazon basin grid cells, from which the tendency of this mean series can exist calculated. Alternatively, the Kendall τ for each band tin be calculated by taking the mean Kendall τ for each private grid jail cell that is within the band. Results from the showtime pick are shown in Figs. 4 and 5 and results from the second method in Supplementary Fig. 15 for AR(1).
The tendencies of the CSD indicators are determined in terms of Kendall τ. This is a rank correlation coefficient with ane variable taken to exist time. Kendall τ values of 1 imply that the fourth dimension serial is always increasing, −1 implies that the time series is ever decreasing and 0 indicates that there is no overall tendency. Following previous piece of work25,52,53, nosotros test the statistical significance of positive tendencies using a test based on phase surrogates that preserve both the variance and the serial correlations of the time series from which the surrogates are constructed. Specifically, we compute the Fourier transform of each fourth dimension series for which we want to exam the significance of Kendall τ, then randomly permute the phases and finally employ the inverse Fourier transform. Since this preserves the ability spectral density, it also preserves the autocorrelation office due to the Wiener–Khinchin theorem. For each fourth dimension serial this procedure is repeated 100,000 times to obtain the surrogates. Kendall τ is computed for each surrogate to obtain the zip model distribution (respective to the supposition of the same variance and autocorrelation simply no underlying trend), from which we calculate a P value by calculating the proportion of surrogates that have a higher Kendall τ value.
Robustness tests
To account for the possibility of man deforestation interfering with the signals we observe (which may not necessarily be detected past the MODIS Country Cover dataset) nosotros also use the Hansen forest loss dataset54 to determine grid cells to remove in an alternate analysis. The original Hansen dataset is at a 0.00025° resolution, 1,000 times higher than the VOD dataset and as such for each VOD grid cell we measure out the pct of Hansen filigree cells that show some forest loss over the fourth dimension period. Note that this dataset does not specify if the observed loss is natural or caused by human deforestation. Excluding whatever VOD filigree cells that contain more than a conservative 5% of lost woods grid cells according to this dataset and running the analysis in the main paper shows like results. Supplementary Figs. xvi–18 are recreations of Figs. 2, iv and v, respectively.
The loss of wood resilience observed as increasing AR(1) in both vegetation indices is supported past another indicator of CSD, namely increasing variance28—of both VOD (Supplementary Fig. 19) and NDVI (Supplementary Fig. twenty). Variance is more than strongly afflicted by changes in the frequency and amplitude of the forcing of a system and as such results could be biased towards individual events. However, nosotros assessed the atmospheric precipitation time serial for changes in variance and found no relationship with the variance signals of VOD and NDVI (Supplementary Fig. 21). Nevertheless, AR(one) is considered the more robust indicator55. Every bit another test of robustness, we partition the grid cells into those that are in floodplains and those that are not (Supplementary Fig. 3). Floodplain data are function of the NASA Large Scale Biosphere–Temper Experiment (LBA-ECO)56. We likewise calculate the resilience signals for the C-ring product of VOD for comparison (Supplementary Fig. 22). Despite the smaller temporal calibration of this product, nosotros still see increases in both AR(1) and variance. To account for a alter in the number of satellites used to calculate VOD, for the Ku-band nosotros also recreate the dataset past sampling a single random solar day per month rather than taking a monthly boilerplate, to mimic a constant satellite pass for the whole time menstruum (Supplementary Fig. 23). Although this expectedly affects the absolute values of AR(ane) and variance, their relative changes over time remain unaffected. To further test the robustness of our results, we looked for similar signals of resilience change in terms of trends in AR(1) in addition to variance in the precipitation time serial used, as a change in the forcing could accept an bear on on the woods that could mistakenly exist interpreted every bit a vegetation resilience loss. However, every bit for the variance, there is no clear increment in the AR(1) of precipitation, nor practise the spatial patterns of both indicators reveal any relationship between irresolute precipitation AR(1) and variance and the observed vegetation resilience loss. Hence, nosotros are confident that changes in precipitation forcing are not driving the vegetation AR(1) signals.
Data availability
Lawmaking availability
All data and lawmaking used for the analysis are available on request from the corresponding author and are published online57.
References
-
Lenton, T. M. et al. Tipping elements in the Earth'southward climate system. Proc. Natl Acad. Sci. U.s. 105, 1786–1793 (2008).
-
Dirzo, R. & Raven, P. H. Global state of biodiversity and loss. Ann. Rev. Environ. Res. 28, 137–167 (2003).
-
Malhi, Y. et al. Climate change, deforestation, and the fate of the Amazon. Science 319, 169–172 (2008).
-
Gatti, L. 5. et al. Amazonia as a carbon source linked to deforestation and climatic change. Nature 595, 388–393 (2021).
-
Brienen, R. J. West. et al. Long-term decline of the Amazon carbon sink. Nature 519, 344–348 (2015).
-
Feldpausch, T. R. et al. Amazon forest response to repeated droughts. Glob. Biogeochem. Cycles xxx, 964–982 (2016).
-
Hubau, West. et al. Asynchronous carbon sink saturation in African and Amazonian tropical forests. Nature 579, eighty–87 (2020).
-
Boers, N., Marwan, North., Barbosa, H. M. J. & Kurths, J. A deforestation-induced tipping betoken for the South American monsoon system. Sci. Rep. 7, 41489 (2017).
-
Cox, P. M., Betts, R. A., Jones, C. D., Spall, S. A. & Totterdell, I. J. Acceleration of global warming due to carbon-cycle feedbacks in a coupled climate model. Nature 408, 184–187 (2000).
-
Cox, P. K. et al. Increasing take a chance of Amazonian drought due to decreasing aerosol pollution. Nature 453, 212–215 (2008).
-
Lovejoy, T. Due east. & Nobre, C. Amazon tipping bespeak. Sci. Adv. 4, eaat2340 (2018).
-
Lovejoy, T. Eastward. & Nobre, C. Amazon tipping bespeak: last adventure for action. Sci. Adv. 5, eaba2949 (2019).
-
Brando, P. M. et al. Sharp increases in Amazonian tree mortality due to drought–fire interactions. Proc. Natl Acad. Sci. USA 111, 6347–6352 (2014).
-
Pueyo, S. et al. Testing for criticality in ecosystem dynamics: the case of Amazonian rainforest and savanna fire. Ecol. Lett. 13, 793–802 (2010).
-
Salati, E., Dall'Olio, A., Matsui, E. & Gat, J. R. Recycling of water in the Amazon basin: an isotopic study. Water Resour. Res. 15, 1250–1258 (1979).
-
Jones, C., Lowe, J., Liddicoat, S. & Betts, R. Committed terrestrial ecosystem changes due to climatic change. Nat. Geosci. 2, 484–487 (2009).
-
Boulton, C. A., Booth, B. B. B. & Good, P. Exploring uncertainty of Amazon dieback in a perturbed parameter Earth system ensemble. Glob. Change Biol. 23, 5032–5044 (2017).
-
Huntingford, C. et al. Simulated resilience of tropical rainforests to COii-induced climate change. Nat. Geosci. half-dozen, 268–273 (2013).
-
Friend, A. D., Stevens, A. M., Knox, R. G. & Cannell, M. G. R. A procedure-based, terrestrial biosphere model of ecosystem dynamics (Hybrid v3.0). Ecol. Model. 95, 249–287 (1997).
-
Poulter, B. et al. Robust dynamics of Amazon dieback to climate change with perturbed ecosystem model parameters. Glob. Change Biol. 16, 2476–2495 (2010).
-
Sitch, S. et al. Evaluation of the terrestrial carbon bicycle, future found geography and climate-carbon cycle feedbacks using five Dynamic Global Vegetation Models (DGVMs). Glob. Change Biol. 14, 2015–2039 (2008).
-
Wissel, C. A universal law of the characteristic return fourth dimension near thresholds. Oecologia 65, 101–107 (1984).
-
Scheffer, M. et al. Early on-alarm signals for disquisitional transitions. Nature 461, 53–59 (2009).
-
Pimm, S. 50. The complication and stability of ecosystems. Nature 307, 321–326 (1984).
-
Dakos, V. et al. Slowing down equally an early warning betoken for abrupt climatic change. Proc. Natl Acad. Sci. U.s. 105, 14308–14312 (2008).
-
Held, H. & Kleinen, T. Detection of climate system bifurcations by degenerate fingerprinting. Geophys. Res. Lett. https://doi.org/10.1029/2004gl020972 (2004).
-
Boulton, C. A., Proficient, P. & Lenton, T. Chiliad. Early on warning signals of simulated Amazon rainforest dieback. Theor. Ecol. vi, 373–384 (2013).
-
Lenton, T. M. Early warning of climate tipping points. Nat. Clim. Change i, 201–209 (2011).
-
Biggs, R., Carpenter, Southward. R. & Brock, W. A. Turning back from the brink: detecting an impending regime shift in time to avert it. Proc. Natl Acad. Sci. USA 106, 826–831 (2009).
-
Boers, N. & Rypdal, M. Critical slowing downwardly suggests that the western Greenland Ice Sail is shut to a tipping point. Proc. Natl Acad. Sci. U.s.a. 118, e2024192118 (2021).
-
Boers, N. Observation-based early-warning signals for a collapse of the Atlantic Meridional Overturning Circulation. Nat. Clim. Modify 11, 680–688 (2021).
-
Verbesselt, J. et al. Remotely sensed resilience of tropical forests. Nat. Clim. Change 6, 1028–1031 (2016).
-
Moesinger, Fifty. et al. The global long-term microwave Vegetation Optical Depth Climate Archive (VODCA). Earth Syst. Sci. Data 12, 177–196 (2020).
-
NOAA Climate Data Record (CDR) of AVHRR Normalized Deviation Vegetation Index (NDVI), Version 5 (NOAA National Centers for Ecology Data, accessed x February 2021); https://doi.org/ten.7289/V5ZG6QH9
-
Liu, Y. Y. et al. Contempo reversal in loss of global terrestrial biomass. Nat. Clim. Change 5, 470–474 (2015).
-
Myneni, R. B., Keeling, C. D., Tucker, C. J., Asrar, Thou. & Nemani, R. R. Increased constitute growth in the northern high latitudes from 1981 to 1991. Nature 386, 698–702 (1997).
-
Friedl, 1000. & Sulla-Menashe, D. MCD12C1, Version 6 (NASA EOSDIS Land Processes DAAC, accessed 20 April 2020); https://doi.org/ten.5067/MODIS/MCD12C1.006
-
Tian, F. et al. Remote sensing of vegetation dynamics in drylands: evaluating vegetation optical depth (VOD) using AVHRR NDVI and in situ light-green biomass data over Due west African Sahel. Remote Sens. Environ. 177, 265–276 (2016).
-
Flores, B. M. et al. Floodplains every bit an Achilles' heel of Amazonian forest resilience. Proc. Natl Acad. Sci. USA 114, 4442–4446 (2017).
-
Funk, C. et al. The climate hazards infrared precipitation with stations—a new environmental record for monitoring extremes. Sci. Data 2, 150066 (2015).
-
Wuyts, B., Champneys, A. R. & House, J. I. Amazonian wood–savanna bistability and human impact. Nat. Commun. 8, 15519 (2017).
-
Fu, R. et al. Increased dry-flavour length over southern Amazonia in recent decades and its implication for future climate projection. Proc. Natl Acad. Sci. United states of america 110, 18110–18115 (2013).
-
Marengo, J. A. et al. Changes in climate and state employ over the Amazon region: current and future variability and trends. Front end. Globe Sci. https://doi.org/10.3389/feart.2018.00228 (2018).
-
Leite-Filho, A. T., Sousa Pontes, V. Y. & Costa, M. H. Furnishings of deforestation on the onset of the rainy flavor and the duration of dry spells in southern Amazonia. J. Geophys. Res. Atmos. 124, 5268–5281 (2019).
-
Leite‐Filho, A. T., Costa, M. H. & Fu, R. The southern Amazon rainy season: the role of deforestation and its interactions with large‐calibration mechanisms. Int. J. Climatol. https://doi.org/10.1002/joc.6335 (2019).
-
Lewis, S. Fifty., Brando, P. 1000., Phillips, O. L., Van Der Heijden, G. 1000. F. & Nepstad, D. The 2010 Amazon drought. Science 331, 554 (2011).
-
Erfanian, A., Wang, M. & Fomenko, L. Unprecedented drought over tropical South America in 2016: significantly under-predicted by tropical SST. Sci. Rep. 7, 5811 (2017).
-
Ciemer, C. et al. An early on-warning indicator for Amazon droughts exclusively based on tropical Atlantic sea surface temperatures. Environ. Res. Lett. 15, 094087 (2020).
-
Rayner, N. A. et al. Global analyses of sea surface temperature, sea water ice, and night marine air temperature since the tardily nineteenth century. J. Geophys. Res. https://doi.org/10.1029/2002jd002670 (2003).
-
Esquivel‐Muelbert, A. et al. Compositional response of Amazon forests to climatic change. Glob. Change Biol. 25, 39–56 (2019).
-
Cleveland, R. B., Cleveland, W. S. & Terpenning, I. STL: a seasonal-trend decomposition procedure based on Loess. J. Off. Stat. six, 3 (1990).
-
Rypdal, Chiliad. Early-warning signals for the onsets of Greenland interstadials and the younger dryas–preboreal transition. J. Clim. 29, 4047–4056 (2016).
-
Boers, N. Early-alarm signals for Dansgaard–Oeschger events in a loftier-resolution water ice core record. Nat. Commun. 9, 2556 (2018).
-
Hansen, 1000. C. et al. High-resolution global maps of 21st-century forest cover alter. Science 342, 850–853 (2013).
-
Dakos, 5., Van Nes, E. H., D'Odorico, P. & Scheffer, M. Robustness of variance and autocorrelation as indicators of critical slowing down. Ecology 93, 264–271 (2012).
-
Hess, L. 50. et al. LBA-ECO LC-07 Wetland Extent, Vegetation, and Inundation: Lowland Amazon Basin (ORNL DAAC, 2005); https://doi.org/x.3334/ORNLDAAC/1284
-
Boulton, C. A. Amazon rainforest resilience data. Zenodo https://doi.org/10.5281/zenodo.5837469 (2022).
Acknowledgements
Nosotros give thanks B. Sakschewski for his helpful comments on the inquiry. N.B. acknowledges funding by the Volkswagen Foundation. This is TiPES contribution no. 107. The TiPES project ('Tipping Points in the Globe System') has received funding from the European Union'south Horizon 2020 inquiry and innovation programme under grant agreement no. 820970. C.A.B. and T.Thou.L. were supported by the Leverhulme Trust (RPG-2018-046). T.Grand.L. was as well supported by a grant from The Alan Turing Institute under a Turing Fellowship (R-EXE-001).
Author information
Affiliations
Contributions
Due north.B. and C.A.B. conceived and designed the study with input from T.M.L. C.A.B. performed the numerical assay with contributions from N.B. All authors discussed and interpreted results, drew conclusions and wrote the paper.
Corresponding writer
Ethics declarations
Competing interests
The authors declare no competing interests.
Peer review
Peer review data
Nature Climate Change thanks Albertus J. Dolman, Bernardo Flores and the other, anonymous, reviewer(s) for their contribution to the peer review of this piece of work.
Boosted information
Publisher's notation Springer Nature remains neutral with regard to jurisdictional claims in published maps and institutional affiliations.
Supplementary data
Rights and permissions
Open Access This article is licensed under a Creative Commons Attribution 4.0 International License, which permits utilize, sharing, accommodation, distribution and reproduction in whatever medium or format, as long as you lot give appropriate credit to the original writer(s) and the source, provide a link to the Creative Commons license, and indicate if changes were fabricated. The images or other third party textile in this article are included in the article's Artistic Eatables license, unless indicated otherwise in a credit line to the fabric. If material is not included in the article'south Creative Commons license and your intended employ is not permitted by statutory regulation or exceeds the permitted use, yous will demand to obtain permission directly from the copyright holder. To view a copy of this license, visit http://creativecommons.org/licenses/by/four.0/.
Reprints and Permissions
Virtually this article
Cite this article
Boulton, C.A., Lenton, T.Grand. & Boers, N. Pronounced loss of Amazon rainforest resilience since the early on 2000s. Nat. Clim. Chang. 12, 271–278 (2022). https://doi.org/10.1038/s41558-022-01287-8
-
Received:
-
Accepted:
-
Published:
-
Upshot Date:
-
DOI : https://doi.org/x.1038/s41558-022-01287-8
Further reading
Source: https://www.nature.com/articles/s41558-022-01287-8
Posted by: davisandessaint.blogspot.com
0 Response to "How Has The Amazon Rainforest Changed Over Time?"
Post a Comment